Appliances
Data Annotation Technology: Empowering AI Development
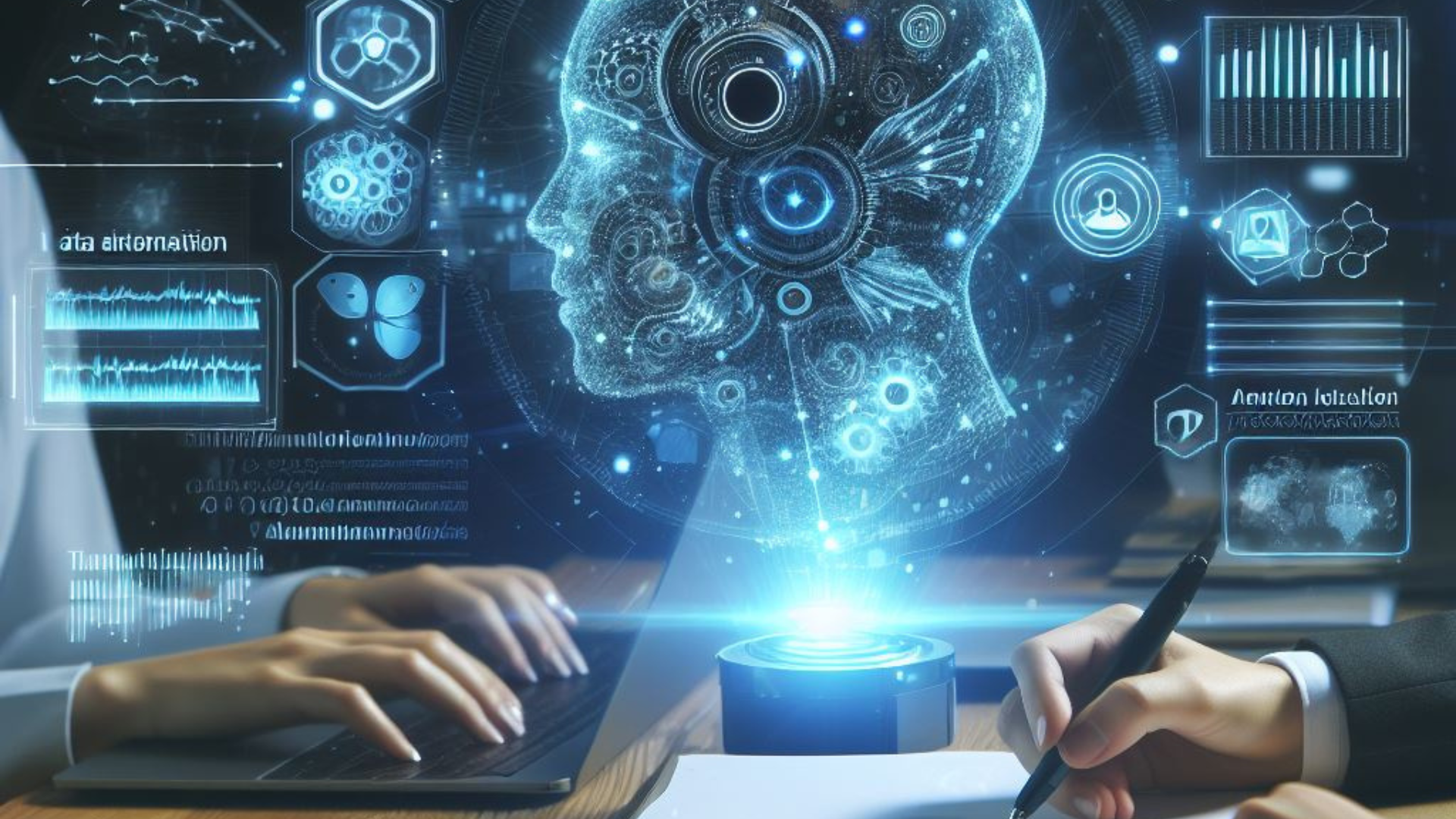
In the age when AI and machine learning are developing, data annotation is at the center of the path from raw data to decisions that are right. Whether it is training autonomous vehicles or powering voice-enabled devices, data labeling serves as the fuel that drives the model development of AI models in a broad range of industries. In this, we shed light on the intricacies of data annotation technology, particularly its role, challenges, tools and prospects.
Briefing about Data Annotation Technology
The annotation of the data implies the labeling or tagging of the data before its reading by the machine. Adding metadata of objects attributes, keywords, or labels to dataset encourages AI tools to perceive patterns, understand insights, and make right decisions. Be it image recognition and natural language processing or prediction and recommendation systems, the data annotation is groundwork on which the AI-based applications are established that are now at the core of our everyday lives.
Understanding Data Annotation
Data annotation, in essence, is a process of adding meta data and labels to unstructured data so that machine learning algorithms can understand the context and meaning of data used as input. Data annotation in AI development is a multifaceted process that encompasses the annotation of multiple data types, such as images, videos, text, and sensor data for training AI models. Bounding box, polygon, keypoints, and semantic segmentation mask are the most commonly used annotation types to differentiate applications and objectives.
High Search Volume Keywords and Their Importance
Keywords with the highest search volume ranking are the main factor to consider regarding the strategic positioning of high visibility content and the possibility to engage a wider audience. Keywords including “data annotation”, “machine learning”, and “AI development” have been seen by search engines as dominating terms which boost the possibility of readers finding the content on data annotation technologies. Selecting appropriate high-volume search-term keywords, therefore, is critical in expanding the reach of the material and thus the essential insights about data annotation are revealed.
Low Search Volume Keywords and their Significance
Although the high search volume keywords are irreplaceable, low search volume ones have the same power in optimal content. These keywords are relatively less common searches that suit the specific niches or long-tail search queries, specifically targeting a sub-segment of audience. For writers to target those looking for in-depth information or even resources that are specific to data annotation, they can go for low search volume keywords like “image annotation techniques” or “text annotation tools”.
Types of Data Annotation Methods
The techniques of data annotation are differentiated by data type and the particular AI application requirements. For image data, image annotation techniques of choice include bounding boxes outlining objects locations, polygons with multiple vertices that delineate object shapes, and semantic segmentation which assigns pixel-level labels to image regions. In the same way, textual data can be labeled as named entities, sentiment scores, or part-of-speech tags to make natural language processing tasks easier.
Challenges in Data Annotation
However, data annotation is not immune to certain challenges such as for annotation teams. Typical obstacles might be data complexity, annotating errors, scalability obstacle and quality control issue. With the increase in the size and abundance of dataset, the annotation teams have to develop efficient workflows, adopt strong quality assurance measures, and make use of automation tools to guarantee unambiguous and consistent annotations.
Tools and Platforms for Data Annotation
A lot of annotation tools and platforms simplify the data annotation process and increase the level of productivity. These tools include annotation software from advanced features to basic annotation software as well as incorporation of machine learning frameworks, real-time feedback, and collaborative annotation. Prominent annotation tools are LabelImg, LabelMe, VGG Image Annotator and Amazon SageMaker Ground Truth, with each offering distinct features to answer a variety of annotation project needs.
Quality Assurance and Validation
It is essential to render the accuracy and harmony of annotations in order to achieve goals of AI projects. A quality of the measures like inter-tagger agreement analysis, cross-checking, and anomaly detection helps identify and dispense of the errors misclassifications in the annotations. Furthermore, the platform provides annotation techniques such as the use test datasets and blind evaluations as additional ways for model performance evaluations and generalization capability validation, which indicates promising data.
Ethical Issues Relating Data Annotation
The ethical issues are right on the horizon in data annotation, including surveillance, bias and fairness. Ethical annotation teams needs to create parameters and adhere to the procedure when it comes to the data preservation and lining up with data principles, avoiding the discrepancies regarding bias or discrimination in AI algorithms. Transparent annotation process, wisely planned informed consent protocols, and bias mitigation measures are main instruments of this kind that help to maintain ethical standards and avoid misuse of annotated data.
Data annotation influence the AI development process
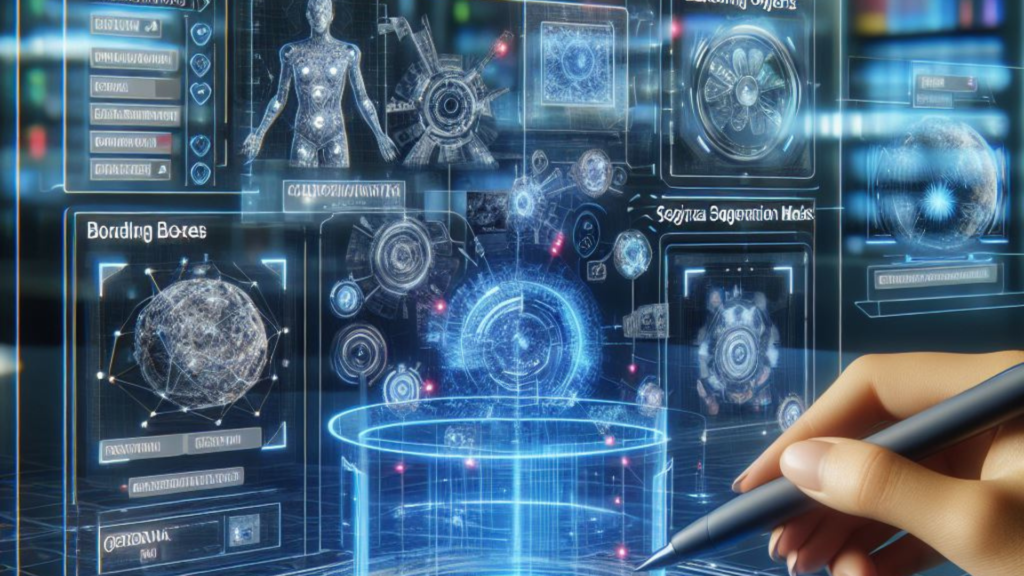
The data annotation stands out as the hub in developing machine learning systems because it supplies the labeled datasets into which training machine learning algorithms enable them to discover the patterns and make the reliable predictions. Top-level annotation helps AI models to assimilate from examples of diverse sources, reuse examples of previously utilized data, and perform well for real-world scenarios. Perceiving the rapid diffusion of AI applications through all industries, it is expected that the demand for the annotated data will rise, which in turn will stimulate the economies of data annotation technologies.
Future Direction in Data Annotation
The future of data annotation technology develops through the takes place which will revolutionize the process and make it more successful. These software applications running on both AI and machine learning algorithms are getting perfected to automate annotation process, minimize human efforts, and increase annotation quality. Technology with advanced active learning, semi-supervised learning, and transfer learning techniques will probably make annotation workflows easier, faster as well, applicability of them for various fields to extend.
Case Studies and Success Stories
Many organizations and research institutions have used annotation technology to create AI projects and they have achieved impactful initiatives. Having AI in healthcare, finance, or even retail and automotive industries can improve the decision-making capabilities, productivity, and innovation for organizations by data annotation. For instance, self-driving car companies utilize labeled datasets to train AI algorithms enabling object detection, lane detection and recognition of traffic signs, heralding a safe self-driving technology.
Training and Education on Data Annotation
Training and education teaching the skills and expertise necessary for data labeling are critically important. Data annotation tools, annotation techniques, and quality assurance practices will all be covered through online courses, workshops, and certification programs, therefore providing people with practical skills. Besides that, the training seminars and resources offered by academic institutions and professional bodies help in ensuring that annotation teams are appropriately equipped to deal with the challenges and complexities that characterize real-world annotation projects.
Industry Perspectives of Data Annotation
Big names in the industry provide the much-desired real life data annotation techniques and their implications for the future of AI development. Through the annotation service providers and AI startups to multinational corporations and research institutions, views of different areas contribute to best practices, innovations, and trends in the data annotation. The collaborative actions and knowledge sharing of different industry players create the culture of innovation and enable the adoption of latest technologies. The product promotion of responsible annotated data utilization in AI applications completes this process.
Conclusion
Data annotation technology becomes a focal point in AI creation enabling machines more comprehension and understanding of the surrounding environment. The addition of labels, annotations, and other forms of embellishment to large datasets is what teams of annotators provide machine learning algorithms with in order to enable them to recognize patterns, draw meaningful conclusions, and make intelligent decisions based on the available data. As AI applications elaborates and delves into unconventional fields, the need for annotated data is rising immensely and this would pioneer innovations, investments, and progress in data annotation technology. With embracing the young trends, outdoing the challenges, and abiding with the code of ethics, we can leverage the potential of data annotation to push the next wave of AI technology and build a promising future for everyone.
Appliances
A World of Opulence: The Most Expensive Mechanical and Smart expensive watches for Men and Women
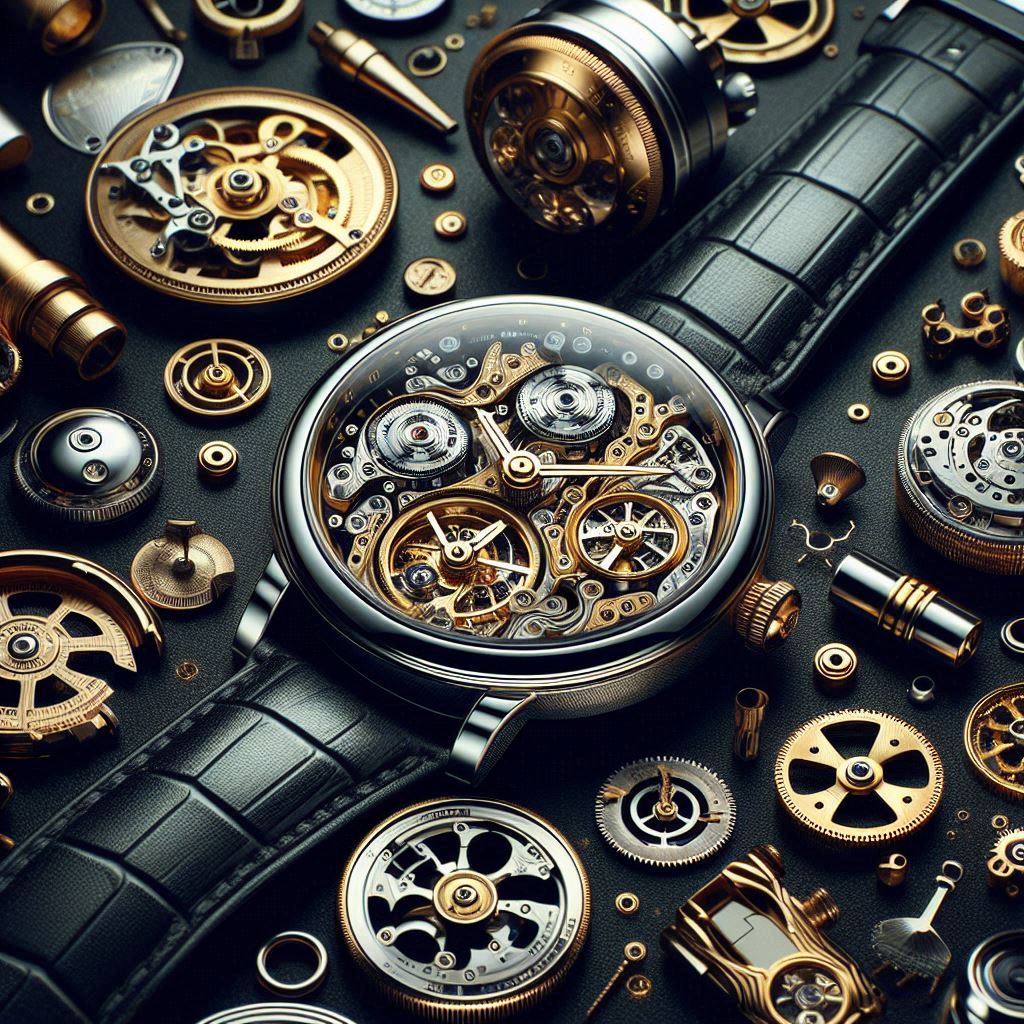
A World of Opulence: The Most Expensive Mechanical and Smart expensive watches for Men and Women Luxury watches pass past mere timekeeping; they’re extremely good statements of workmanship, technology, and style. This article delves into the world’s maximum high-priced mechanical and smart watches, showcasing ten masterpieces for each males and females in every elegance. From the problematic mechanics of mechanical watches to the contemporary-day era of smartwatches, we discover the attraction, features, and unique developments that make those timepieces stand out within the realm.
Mechanical Marvels: High-End Timepieces for Men expensive watches
- Patek Philippe Grandmaster Chime Ref. 6300A-010
Price: ~$31 Million
Specifications:
Movement: Caliber three hundred Grand Complication
Functions: 20 complications including a grand strike, minute repeater, and Westminster chime
Case: Stainless metallic with a duration of forty seven.7 mm
Dial: Reverse hand-engraved with a silvery opaline finish
Power Reserve: Approximately seventy two hours
Features:
The Patek Philippe Grandmaster Chime Ref. 6300A-010 is the undisputed king of steeply-priced watches, retaining the report for the most pricey wristwatch ever sold at public sale. This timepiece features 20 complications, such as a grand strike with a minute repeater, a perpetual calendar, and a Westminster chime. The hard hand-engraved dial, coupled with the chrome steel case, showcases Patek Philippe’s mastery in blending conventional craftsmanship with progressive engineering. This watch is not only a timekeeper but a symphony of horological genius, making it a coveted gem for creditors worldwide.
- A. Lange & Söhne Double Split
Price: ~$1.Three Million
Specifications:
Movement: Caliber L001.2, manually wound
Functions: Split-seconds chronograph, perpetual calendar, moon section
Case: Pink gold, diameter of 41.Nine mm
Dial: Silvered dial with black engraved numerals
Power Reserve: Approximately 55 hours
Features:
The A. Lange & Söhne Double Split is a masterpiece that combines sophisticated layout with technical prowess. It boasts a break up-seconds chronograph, a perpetual calendar, and a moon phase complication. Housed in a beautiful purple gold case, its silvered dial with black engraved numerals enhances its traditional appeal. The double split function lets in for simultaneous time measurements, making it a favorite among severe watch connoisseurs. This watch exemplifies the logo’s determination to precision and beauty, making it a image of reputation and sophistication.
- Richard Mille RM fifty seven-03 Tourbillon Diamond Twister
Price: ~$820,000
Specifications:
Movement: RM 57-03 manual winding, tourbillon
Functions: Hours, mins, tourbillon
Case: Titanium and sapphire crystal with diamonds
Dial: Sapphire crystal with skeletonized tourbillon
Power Reserve: Approximately 50 hours
Features:
The Richard Mille RM fifty seven-03 Tourbillon Diamond Twister is a surprise of engineering and layout. Its innovative crown rotates 100 and twenty levels to wind the motion, a function that devices it other than traditional watches. The case is adorned with diamonds, showcasing Richard Mille’s aptitude for combining opulence with technical innovation. The skeletonized dial offers a clear view of the complex tourbillon movement, emphasizing the watch’s avant-garde attraction. This timepiece isn’t most effective a testomony to Richard Mille’s craftsmanship but also a photo of futuristic design and comfort.
Four. Vacheron Constantin Tour de l’Ile
Price: ~$1.Five Million
Specifications:
Movement: Caliber 2755, manual winding
Functions: 26 headaches, which includes perpetual calendar, sky chart, and equation of time
Case: Platinum, diameter of forty seven mm
Dial: Silvered opaline with guilloché decoration
Power Reserve: Approximately fifty eight hours
Features:
The Vacheron Constantin Tour de l’Ile is a masterpiece of watchmaking with 26 complications, making it one of the maximum complex wristwatches inside the international. It capabilities a perpetual calendar, a sky chart, and an equation of time, among other capabilities. The platinum case with a diameter of 47 mm and the silvered opaline dial with guilloché decoration highlight its expensive format. This watch is a actual engineering marvel, blending artistry with horological innovation. The Tour de l’Ile is a party of Vacheron Constantin’s historical past and understanding in developing first-rate timepieces.
- Roger Dubuis Excalibur Double Flying Tourbillon
Price: ~$390,000
Specifications:
Movement: RD01SQ, manually wound
Functions: Hours, mins, double flying tourbillon
Case: forty five mm titanium with purple gold factors
Dial: Skeletonized with pink gold and black accents
Power Reserve: Approximately 48 hours
Features:
The Roger Dubuis Excalibur Double Flying Tourbillon is a actual feat of horological engineering. It features two tourbillons that negate the consequences of gravity on the motion, improving its accuracy. The skeletonized dial with purple gold and black accents offers a fascinating view of the complicated mechanism. Housed in a forty five mm titanium case with pink gold elements, this watch exudes a ambitious, avant-garde aesthetic. The Excalibur Double Flying Tourbillon is a testament to Roger Dubuis’s dedication to pushing the bounds of watchmaking.
- Greubel Forsey Art Piece Edition 1
Price: ~$1.5 Million
Specifications:
Movement: Caliber GF01, manually wound
Functions: Three-dimensional tourbillon, round differential, and planetary tools
Case: 47.5 mm platinum with sapphire crystal
Dial: Sapphire crystal with 3-dimensional tourbillon
Power Reserve: Approximately seventy hours
Features:
The Greubel Forsey Art Piece Edition 1 is a masterpiece of contemporary watchmaking, offering a three-dimensional tourbillon, a spherical differential, and a planetary tools machine. The 47.Five mm platinum case with sapphire crystal presents a striking view of the motion. The sapphire crystal dial complements the transparency, allowing the difficult mechanics to be completely preferred. This watch is a mix of art work and technological know-how, showcasing Greubel Forsey’s innovative spirit and dedication to pushing the boundaries of horological design.
- Audemars Piguet Royal Oak Offshore Grande Complication
Price: ~$1.Eight Million
Specifications:
Movement: Caliber 2889, manual winding
Functions: Minute repeater, perpetual calendar, chronograph
Case: 44 mm platinum with rubber and gold accents
Dial: Black with blue accents and skeletonized fingers
Power Reserve: Approximately 45 hours
Features:
The Audemars Piguet Royal Oak Offshore Grande Complication is a stunning mixture of sportiness and sophistication. It functions a minute repeater, a perpetual calendar, and a chronograph, all housed in a forty four mm platinum case with rubber and gold accents. The black dial with blue accents and skeletonized fingers gives to its ambitious, current look. This watch is a image of Audemars Piguet’s legacy in developing ultra-modern, excessive-performance timepieces that integrate conventional craftsmanship with modern format.
Eight. Hublot Big Bang Tourbillon
Price: ~$500,000
Specifications:
Movement: HUB6010, guide winding
Functions: Hours, minutes, tourbillon
Case: 45 mm titanium with ceramic bezel
Dial: Skeletonized with black and crimson accents
Power Reserve: Approximately a hundred and twenty hours
Features:
The Hublot Big Bang Tourbillon is a super fusion of technology and layout. It features a manually wound HUB6010 motion with a tourbillon, housed in a 45 mm titanium case with a ceramic bezel. The skeletonized dial with black and red accents gives a dramatic view of the tourbillon mechanism. This watch is a testomony to Hublot’s innovation in blending materials and mechanics, creating a timepiece this is each beneficial and visually lovely.
- Breguet Classique Tourbillon Ref. 5377
Price: ~$three hundred,000
Specifications:
Movement: Caliber 581, manually wound
Functions: Tourbillon, hours, minutes
Case: forty one mm platinum
Dial: Silvered gold with hand-engraved information
Power Reserve: Approximately eighty hours
Features:
The Breguet Classique Tourbillon Ref. 5377 is a classic example of Breguet’s determination to standard watchmaking. It talents a manually wound Caliber 581 motion with a tourbillon, housed in a forty one mm platinum case. The silvered gold dial with hand-engraved info showcases Breguet’s signature fashion and craftsmanship. This watch is a celebration of Breguet’s wealthy history, providing an excellent mixture of beauty, precision, and undying beauty.
- F.P. Journe Chronomètre à Résonance
Price: ~$3 hundred,000
Specifications:
Movement: Caliber 1499.Three, manually wound
Functions: Hours, minutes, chronometer with resonance
Case: 40 mm platinum
Dial: Silvered gold with guilloché ornament
Power Reserve: Approximately forty hours
Features:
The F.P. Journe Chronomètre à Résonance is a masterpiece of precision and innovation. It capabilities a manually wound Caliber 1499.Three movement with a resonance chronometer, housed in a forty mm platinum case. The silvered gold dial with guilloché ornament presentations F.P. Journe’s dedication to conventional craftsmanship and aesthetic refinement. This watch is renowned for its precise resonance mechanism, which enhances accuracy with the resource of synchronizing the vibrations of stability wheels. The Chronomètre à Résonance exemplifies F.P. Journe’s pursuit of horological excellence and technical ingenuity.
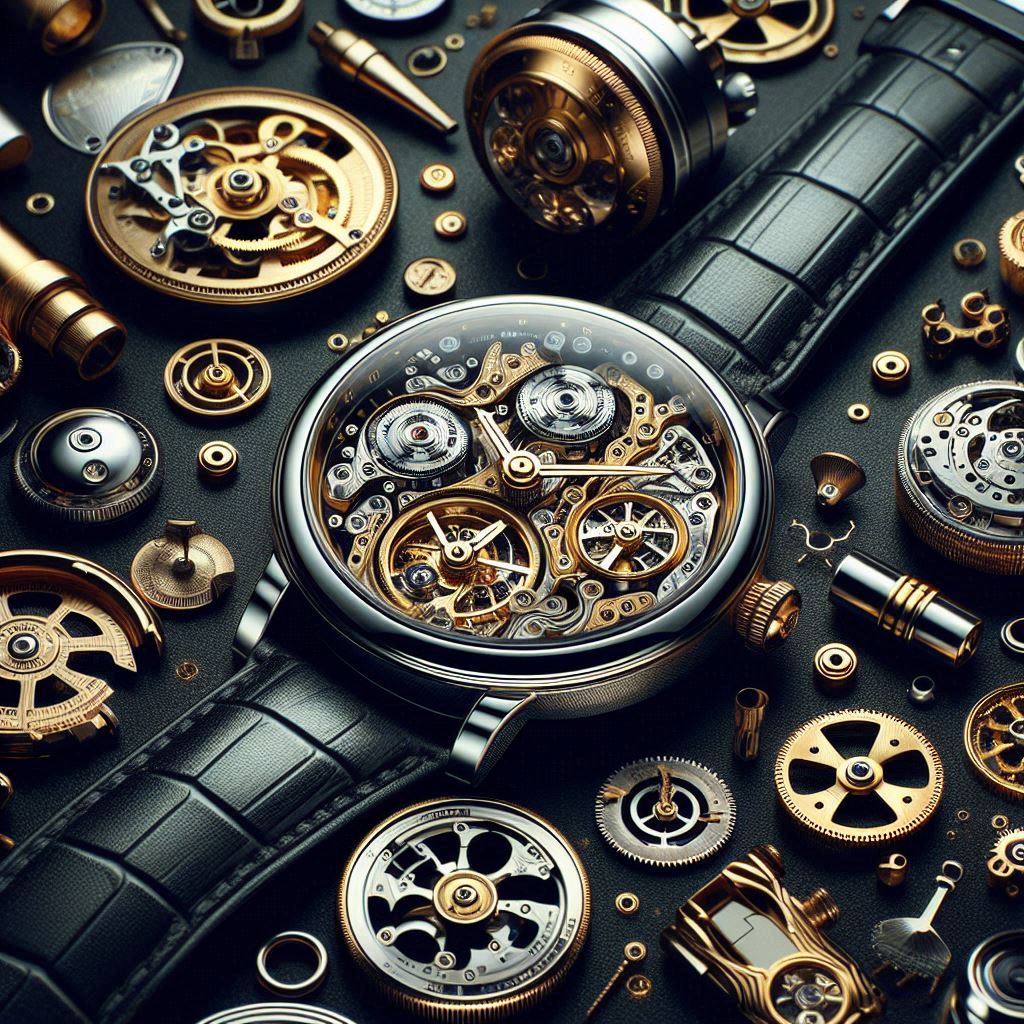
For the Ladies: Exquisite Mechanical Wristwatches
- Cartier Tortue de Formes Phoenix
Price: ~$1.Four Million
Specifications:
Movement: Caliber 9602 MC, guide winding
Functions: Hours, mins
Case: Platinum with gemstones and feathers
Dial: Silvered opaline with guilloché decoration
Power Reserve: Approximately 48 hours
Features:
The Cartier Tortue de Formes Phoenix is a real masterpiece of artistry and engineering. Its platinum case is formed like a phoenix, embellished with colorful gems and feathers. The silvered opaline dial with guilloché decoration affords to its pricey enchantment. This watch is a testament to Cartier’s statistics in combining high earrings with outstanding watchmaking, growing a timepiece that is as lovable as it’s miles state-of-the-art. The Tortue de Formes Phoenix is a rare gem that embodies Cartier’s legacy of beauty and innovation.
- Van Cleef & Arpels Heures d’Elles Poetic Complications
Price: ~$230,000
Specifications:
Movement: Caliber 138, automatic winding
Functions: Hours, minutes, retrograde date, moon stages
Case: White gold with diamonds
Dial: Mother-of-pearl with enamel butterfly and bird motifs
Power Reserve: Approximately 40 hours
Features:
The Van Cleef & Arpels Heures d’Elles Poetic Complications is a party of femininity and resourceful expression. Its white gold case is decorated with diamonds, growing a shimmering backdrop for the mom-of-pearl dial. The dial functions tooth butterfly and chook motifs that indicate the time and display retrograde date and moon stages. This watch is a testament to Van Cleef & Arpels’ mastery in creating poetic headaches that integrate aesthetic splendor with technical precision. The Heures d’Elles Poetic Complications is a real work of art that captures the essence of time via captivating layout.
Three. Breguet Reine de Naples 8998
Price: ~$one hundred and 80,000
Specifications:
Movement: Caliber 537/1, automatic winding
Functions: Hours, minutes, day-night time indicator, moon phase
Case: White gold with diamonds, 36.Five x 28.Forty five mm
Dial: Natural white mother-of-pearl, hand-engraved with floral motifs
Power Reserve: Approximately 45 hours
Features:
The Breguet Reine de Naples 8998 is a symbol of timeless elegance and class. Its oval case in white gold is embellished with diamonds, including to its high-priced attraction. The natural white mother-of-pearl dial is hand-engraved with sensitive floral motifs, showcasing Breguet’s interest to element and craftsmanship. This watch capabilities a day-night time indicator and a moon phase hardship, combining practical functionality with brilliant design. The Reine de Naples 8998 is a tribute to Queen Caroline Murat of Naples, who stimulated Abraham-Louis Breguet to create the first wristwatch, making it a image of Breguet’s rich background and innovation.
Four. Audemars Piguet Millenary Minute Repeater
Price: ~$four hundred,000
Specifications:
Movement: Caliber 2928, manual winding
Functions: Hours, minutes, minute repeater
Case: 47 mm pink gold with sapphire crystal
Dial: Skeletonized with pink gold hands
Power Reserve: Approximately 48 hours
Features:
The Audemars Piguet Millenary Minute Repeater is a masterpiece of watchmaking craftsmanship. It capabilities a minute repeater problem housed in a forty seven mm purple gold case with sapphire crystal, imparting a glimpse into the complex mechanics of the movement. The skeletonized dial with crimson gold palms complements its present day-day aesthetic while showcasing Audemars Piguet’s commitment to blending way of life with innovation. This watch is a testomony to the emblem’s know-how in growing immoderate-performance timepieces that enchantment to every connoisseurs and creditors.
Five. Jaeger-LeCoultre Rendez-Vous Celestial
Price: ~$a hundred fifty,000
Specifications:
Movement: Caliber 809/1, automated winding
Functions: Hours, mins, celestial problem
Case: White gold with diamonds, 36 mm
Dial: Blue aventurine with diamond-set constellations
Power Reserve: Approximately 40 hours
Features:
The Jaeger-LeCoultre Rendez-Vous Celestial is a celestial marvel that mixes horological artistry with astronomical precision. Its white gold case is decorated with diamonds, including a touch of brilliance to its fashionable format. The blue aventurine dial depicts the night time sky, with diamond-set constellations that tune the passage of time. This watch capabilities Jaeger-LeCoultre’s automatic Caliber 809/1 movement, supplying specific timekeeping and celestial indicators. The Rendez-Vous Celestial is a tribute to the splendor of the cosmos, making it a timeless companion for those who recognize each art and technological expertise.
The Tech Touch: Luxurious Smartwatches
For Her:
- Louis Vuitton Tambour Horizon Light Up expensive watches
Price: ~$3,three hundred
Specifications:
Operating System: Wear OS with the aid of Google
Processor: Qualcomm Snapdragon Wear 3100
Display: 1.3″ AMOLED with mother-of-pearl and customizable straps
Connectivity: Wi-Fi, Bluetooth, GPS
Case: Stainless metal with diamonds, 35 mm
Features: Notifications, activity monitoring, Louis Vuitton distinct watch faces
Features:
The Louis Vuitton Tambour Horizon Light Up smartwatch combines luxurious with current era. It features a 1.Three” AMOLED display with a mother-of-pearl dial and customizable straps, taking into account personalized style. Powered with the aid of manner of Wear OS with the aid of Google and Qualcomm Snapdragon Wear 3100, it offers seamless connectivity with Wi-Fi, Bluetooth, and GPS skills. The stainless-steel case with diamonds gives a hint of beauty, making it a statement piece for fashion-in advance human beings. With specific Louis Vuitton watch faces and vital clever features like notifications and hobby monitoring, the Tambour Horizon Light Up smartwatch is a mixture of favor and capability.
- Gucci Serena Sport expensive watches
Price: ~$1,300
Specifications:
Operating System: Wear OS via Google
Processor: Qualcomm Snapdragon Wear 3100
Display: 1.39″ AMOLED with customizable straps
Connectivity: Wi-Fi, Bluetooth, GPS
Case: Stainless steel with interchangeable straps
Features: Fitness tracking, heart rate monitoring, Gucci distinctive watch faces
Features:
The Gucci Serena Sport smartwatch is a fusion of sporty beauty and Italian luxurious. It talents a 1.39″ AMOLED display with customizable straps, taking into consideration a customized contact. Powered by way of the use of Wear OS via Google and Qualcomm Snapdragon Wear 3100, it ensures smooth overall performance and connectivity with Wi-Fi, Bluetooth, and GPS competencies. The chrome steel case with interchangeable straps offers versatility, at the same time as Gucci’s special watch faces add a wonderful aptitude. With advanced functions like health monitoring and coronary heart price monitoring, the Serena Sport smartwatch is designed for people who prioritize each style and capability of their accessories.
- Hermès Apple Watch Series 8
Price: Varies relying on version and band expensive watches
Specifications:
Operating System: watchOS
Processor: Apple S8 chip
Display: Retina LTPO OLED, sizes range
Connectivity: Wi-Fi, Bluetooth, GPS
Case: Stainless steel or titanium with Hermès leather-based bands
Features: Apple Pay, Siri, Hermès one of a type watch faces
Features:
The Hermès Apple Watch Series eight combines modern-day technology with undying Parisian beauty. Available in severa models and bands made from superb leathers, it gives a customizable revel in that blends seamlessly into any cloth wardrobe. Powered by means of the use of the Apple S8 chip and watchOS, it offers you speedy performance and a responsive person interface. The Retina LTPO OLED show offers colourful visuals, whilst features like Apple Pay and Siri enhance comfort. Hermès one of a kind watch faces add a touch of sophistication, making the Apple Watch Series eight a assertion of highly-priced and innovation.
- Misfit Vapor 2 Stella McCartney
Price: ~$3 hundred
Specifications:
Operating System: Wear OS by using the use of Google
Processor: Qualcomm Snapdragon Wear 2100
Display: 1.2″ AMOLED with customizable straps
Connectivity: Wi-Fi, Bluetooth, GPS
Case: Aluminum with interchangeable straps
Features: Heart rate tracking, hobby tracking, Misfit one-of-a-kind watch faces
Features:
The Misfit Vapor 2 Stella McCartney smartwatch is a collaboration among Misfit and fashion icon Stella McCartney. It combines style-forward layout with essential health and well being features, making it a versatile accent for everyday use. Powered via Wear OS through Google and Qualcomm Snapdragon Wear 2100, it guarantees smooth performance and connectivity with Wi-Fi, Bluetooth, and GPS capabilities. The aluminum case with interchangeable straps gives sturdiness and fashion, at the equal time as Misfit’s one of a type watch faces cater to character tastes. Whether tracking fitness dreams or staying related on the cross, the Vapor 2 Stella McCartney smartwatch blends function with fashion.
Five. Bvlgari Diva’s Dream smart expensive watches
Price: ~$6,800
Specifications:
Operating System: Wear OS through way of Google
Processor: Qualcomm Snapdragon Wear 3100
Display: 1.19″ AMOLED with ceramic and diamonds
Connectivity: Wi-Fi, Bluetooth, GPS
Case: Ceramic with diamond accents, 37 mm
Features: Notifications, interest tracking, Bvlgari unique watch faces
Features:
The Bvlgari Diva’s Dream smartwatch is a jewel at the wrist, combining costly with cutting-edge technology. Crafted from ceramic and embellished with diamonds, its aesthetic attraction is unprecedented. Powered via Wear OS through Google and Qualcomm Snapdragon Wear 3100, it gives seamless connectivity with Wi-Fi, Bluetooth, and GPS competencies. The ceramic case with diamond accents exudes sophistication, making it a declaration piece for discerning individuals. With important smart capabilities like notifications and interest tracking, in addition to Bvlgari’s one in every of a type watch faces, the Diva’s Dream smartwatch is a picture of beauty and innovation.
Conclusion
This article has explored the area of opulent timepieces, from the complicated mechanics of high-stop mechanical watches to the present day technology of costly smartwatches. Each timepiece featured embodies craftsmanship, innovation, and fashion, catering to the discerning tastes of watch aficionados global. Whether you’re captivated through the tricky headaches of a mechanical masterpiece or the seamless integration of technology in a smartwatch, those watches provide a glimpse into the arena of haute horlogerie. Each watch tells a tale of lifestyle, innovation, and timeless splendor, making it not most effective a timekeeper however a chunk of artwork.
Appliances
Exploring the Most expensive smartphones in the World
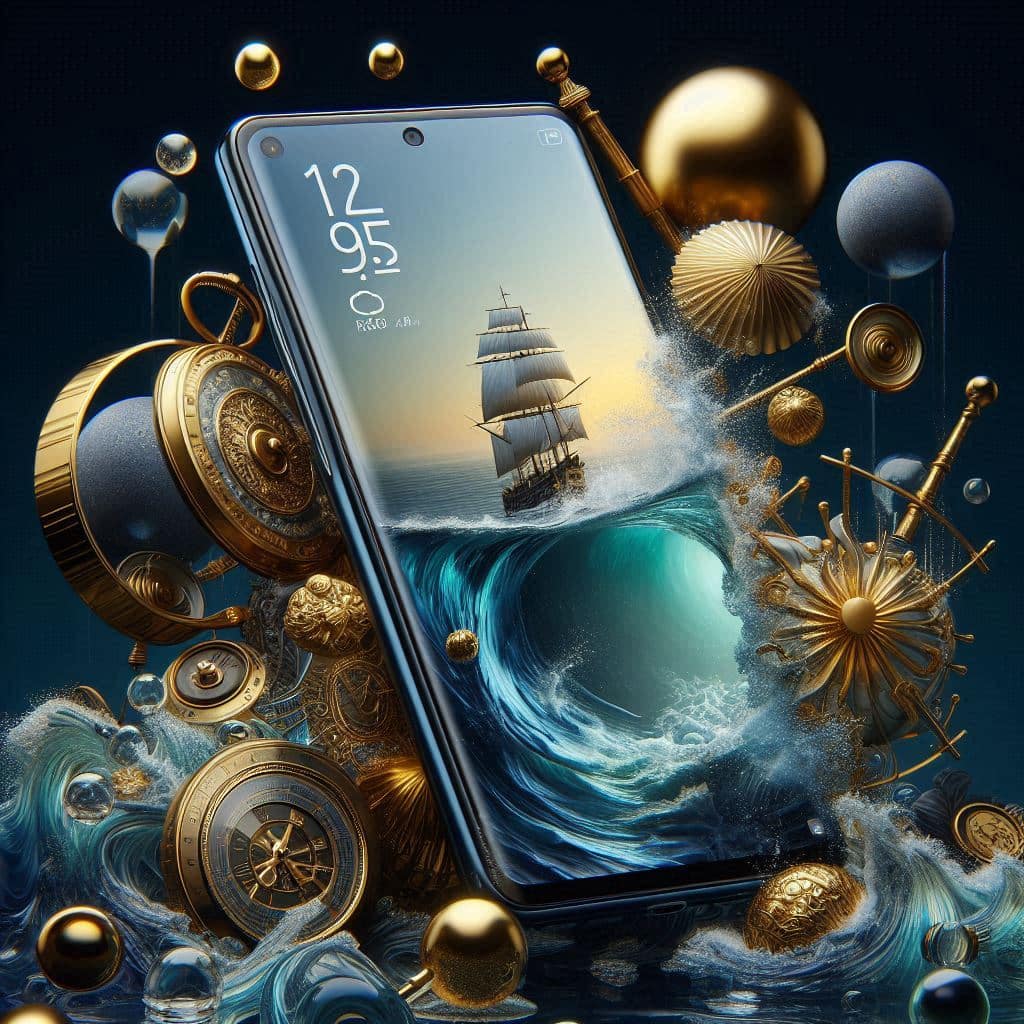
In modern-day interconnected world, expensive smartphones have transcended their utilitarian origins to become symbols of reputation, luxurious, and modern generation. This article embarks on a comprehensive journey through the realm of the arena’s priciest smartphones, meticulously examining their talents, specs, and the huge worldwide carrier networks that guide them. Whether you’re a tech fanatic, a connoisseur of luxury, or virtually interested in the top of cell innovation, this manual will immerse you within the complicated information of the most extravagant smartphones available today.
Defining Luxury expensive smartphones in Mobile Phones
Luxury inside the realm of expensive smartphones is an amalgamation of functionality, remarkable layout, top rate materials, and notable features tailor-made for the discerning few. It represents a mix of technological prowess and artisanal craftsmanship, in which manufacturers spare no cost in pushing the boundaries of what a cellphone can reap. These gadgets are not just equipment; they are statements of opulence and sophistication, catering completely to people who demand not anything but the excellent.
Luxury smartphones are characterized through their exceptional materials and meticulous craftsmanship. Materials along with aerospace-grade titanium, ceramic, carbon fiber, or even valuable metals like gold and platinum are normally used. These materials now not best beautify the durability of the devices however also lend a completely unique aesthetic attraction, placing them aside from mainstream smartphones. Designers pay meticulous interest to element, from the ergonomic contours that match perfectly in hand to the tricky engravings and custom finishes that mirror the owner’s non-public fashion.
The Evolution of Luxury Phones Across Decades
The concept of luxurious telephones has advanced appreciably over the decades, reflecting modifications in technology, purchaser options, and global monetary tendencies. Early luxurious telephones pioneered by using manufacturers like Vertu and Goldvish showcased extravagant designs crafted from materials which includes gold, silver, and special leathers. These devices were characterised not most effective with the aid of their top rate build nice however additionally by means of their one of a kind services tailor-made for affluent shoppers.
As technology stepped forward, luxurious expensive smartphones commenced integrating contemporary features alongside costly materials. This evolution reflected broader developments in purchaser electronics, in which smartphones have become extra than simply conversation devices but additionally repute symbols. The transition from simple capability to excessive-performance computing machines underscored a shift toward blending opulence with technological innovation.
Pioneers within the Luxury Phone Market
The adventure into luxury expensive smartphones started out with trailblazers like Vertu, based in 1998 as a subsidiary of Nokia. Vertu fast established itself via presenting hand made phones embellished with valuable metals and gem stones, coupled with a concierge carrier that catered to the whims of its rich purchasers. Each Vertu smartphone was a testament to bespoke luxury, combining traditional craftsmanship with cutting-edge era.
Similarly, Goldvish emerged as another pioneer, that specialize in phones embellished with diamonds and uncommon materials. Founded in Switzerland, Goldvish epitomized European craftsmanship and exclusivity, appealing to creditors and aficionados in search of one-of-a-kind luxury devices. These early innovators set the stage for the convergence of luxurious and technology in the cell phone industry.
Modern Era: Integrating Technology with Luxury expensive smartphones
In current years, mainstream expensive smartphones giants which include Apple, Samsung, and Huawei have ventured into the posh phase, offering devices that blend state-of-the-art era with premium materials. This shift has democratized luxurious telephones to a point, making excessive-quit capabilities more available whilst preserving exclusivity through restrained variations and bespoke customization alternatives.
Luxury variations of famous smartphones regularly characteristic stronger specifications, one-of-a-kind finishes, and personalised offerings that cater to the tastes of affluent purchasers. These gadgets now not handiest showcase the ultra-modern improvements in cell era however additionally spotlight the craftsmanship involved of their design and manufacturing. From bespoke software interfaces to custom-designed accessories, luxurious smartphones redefine personalization in consumer electronics.
Features and Specifications of the Most Expensive Phones expensive smartphones
When exploring the arena’s maximum pricey expensive smartphones, every tool represents a marvel of engineering, design, and technological innovation. These devices pass beyond conventional smartphones, supplying capabilities that redefine person expectancies and set new benchmarks within the industry.
Advanced Display Technologies
The display serves as a focal point for luxury expensive smartphones, presenting advancements along with OLED (Organic Light-Emitting Diode) and AMOLED (Active Matrix Organic Light-Emitting Diode) technology. These presentations offer colourful colorings, deep blacks, and power efficiency, enhancing both visible experiences and battery existence. Some luxury telephones even include bendy display technologies that curve elegantly across the device, similarly raising their aesthetic attraction.
State-of-the-Art Camera Systems
The photography talents of luxurious expensive smartphones rival those of professional cameras, thanks to excessive-resolution sensors, advanced optics, and complicated photograph processing algorithms. Multi-lens setups, including telephoto, extensive-angle, and macro lenses, enable users to capture designated shots in numerous lighting conditions. Artificial Intelligence (AI) upgrades similarly optimize snap shots, making sure first rate readability and realism in every photo.
Exceptional Performance expensive smartphones
Luxury telephones prioritize overall performance with the state-of-the-art-generation processors, enough RAM (Random Access Memory), and expansive storage options. These specifications not most effective assist annoying programs like gaming and multimedia editing however also ensure a seamless person experience. High-performance portraits processors and AI accelerators beautify gaming and augmented reality (AR) stories, making luxury phones flexible tools for both productivity and leisure.
Design Excellence and Premium Materials
Crafted from substances inclusive of aerospace-grade titanium, ceramic, and scratch-resistant sapphire glass, luxurious phones combine sturdiness with elegance. Designers meticulously refine each thing of those gadgets, from ergonomic contours to tricky floor finishes, developing works of artwork that resonate with luxurious and sophistication. Custom finishes, along with hand-polished metallic frames and leather-wrapped backs, mirror a commitment to craftsmanship and exclusivity.
Global Service Networks
Owning a luxurious expensive smartphones includes more than possessing a premium device; it involves get entry to to a complete assist and provider ecosystem that spans continents. Manufacturers apprehend the significance of worldwide reach, ensuring that customers acquire spark off assistance and customized care wherever they will be placed.
Service Excellence Across Continents
In North America, luxurious telephone provider centers are strategically positioned in essential metropolitan regions, providing personalized assist and expedited restore offerings. Brands prioritize client pleasure through offering concierge offerings for VIP customers, ensuring that their gadgets acquire meticulous attention and care.
European Heritage of Craftsmanship
Europe boasts a rich heritage of craftsmanship, meditated in the meticulous provider supplied for luxurious telephones. Service centers combination conventional expertise with present day diagnostics, making certain that every device is maintained to the highest requirements. Whether repairing difficult hardware or updating bespoke software program functions, European carrier facilities uphold a legacy of excellence and attention to element.
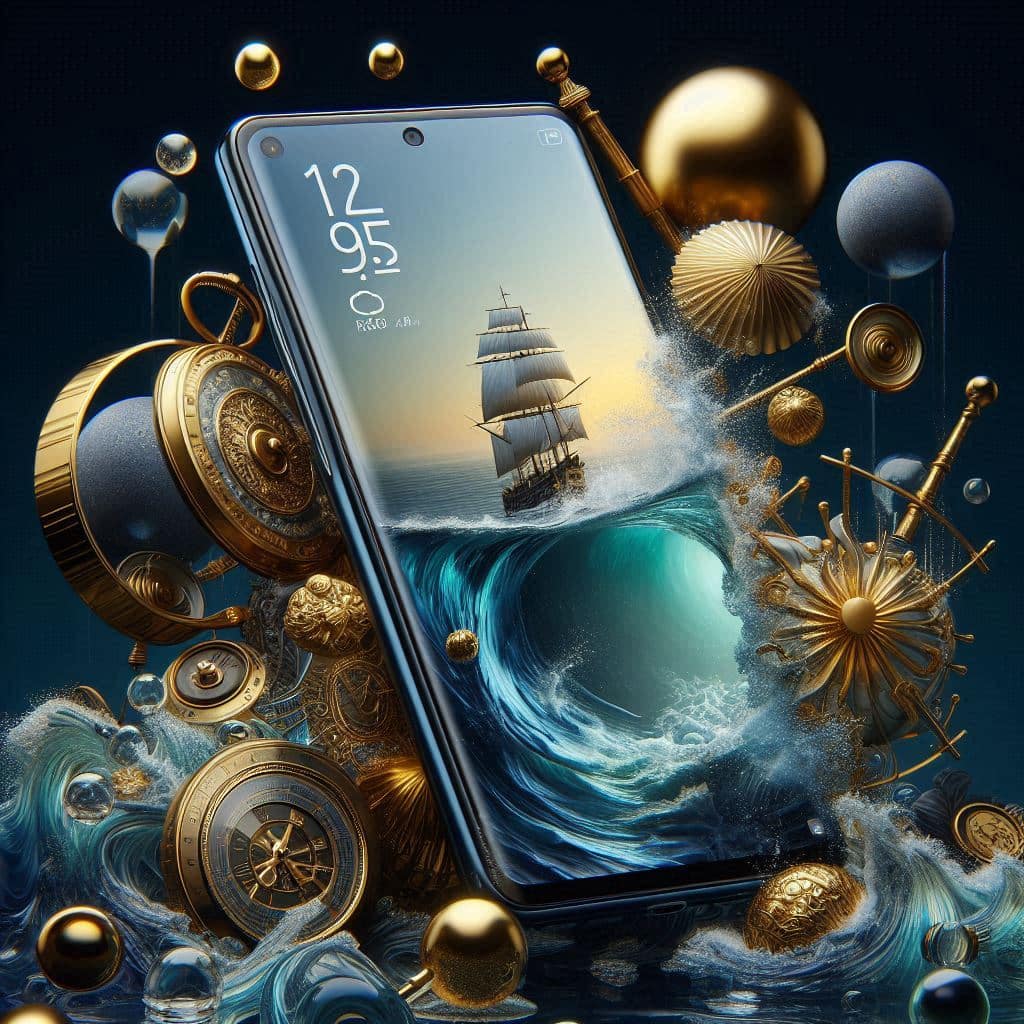
Technological Advancements in Asia-Pacific
Asia-Pacific serves as a hub of technological innovation, with luxurious cellphone carrier centers equipped with ultra-modern diagnostic gear and specialized know-how. Customers advantage from efficient carrier methods and access to specific add-ons, making sure that their gadgets remain at the forefront of generation and style.
Tailored Services inside the Middle East and Africa
Service centers within the Middle East and Africa cater to the specific options of luxurious telephone owners, supplying bespoke answers and personalized help. Concierge offerings are not unusual, presenting VIP customers with unique privileges and ensuring that their luxurious devices maintain their prestige and capability.
Future Trends in Luxury Phones expensive smartphones
As generation continues to advance and purchaser expectancies evolve, the panorama of luxurious phones will absolutely undergo further modifications. Manufacturers will innovate no longer most effective in phrases of features and substances but also within the provider stories they offer globally. Enhanced connectivity, augmented fact integration, and sustainable manufacturing practices are probable to form the destiny of luxurious smartphones, catering to a discerning target market that values innovation, exclusivity, and environmental stewardship.
Luxury smartphones are also possibly to include advanced security features inclusive of biometric authentication and stable hardware modules to shield touchy data. These gadgets will increasingly integrate seamlessly into smart home ecosystems, supplying more advantageous comfort and connectivity.
Furthermore, the customization options for luxury smartphones are anticipated to expand, allowing users to personalize no longer most effective the outside design however additionally the software program interface and capability. Brands may also collaborate with renowned artists, designers, and luxury brands to create constrained-edition collections that cater to precise aesthetic tastes and cultural developments.
In Conclusion
The global’s maximum high priced phones epitomize the convergence of cell innovation and comfort. From their contemporary functions to their tremendous worldwide service networks, those devices cater to folks who are searching for remarkable great, craftsmanship, and exclusivity in every aspect of their cellular revel in. Whether as symbols of prestige or as gear for productivity and entertainment, luxurious smartphones continue to redefine the limits of what is possible in purchaser electronics, setting new standards for innovation and elegance in the virtual age.
-
Harnessing Solar Power On-the-Go: The Rise of Foldable Solar Panel Installations
-
A World of Opulence: The Most Expensive Mechanical and Smart expensive watches for Men and Women
-
Exploring the Most expensive smartphones in the World
-
Introduction to Network Cards: Evolution, Types, and Technologies
Appliances
Introduction to Network Cards: Evolution, Types, and Technologies
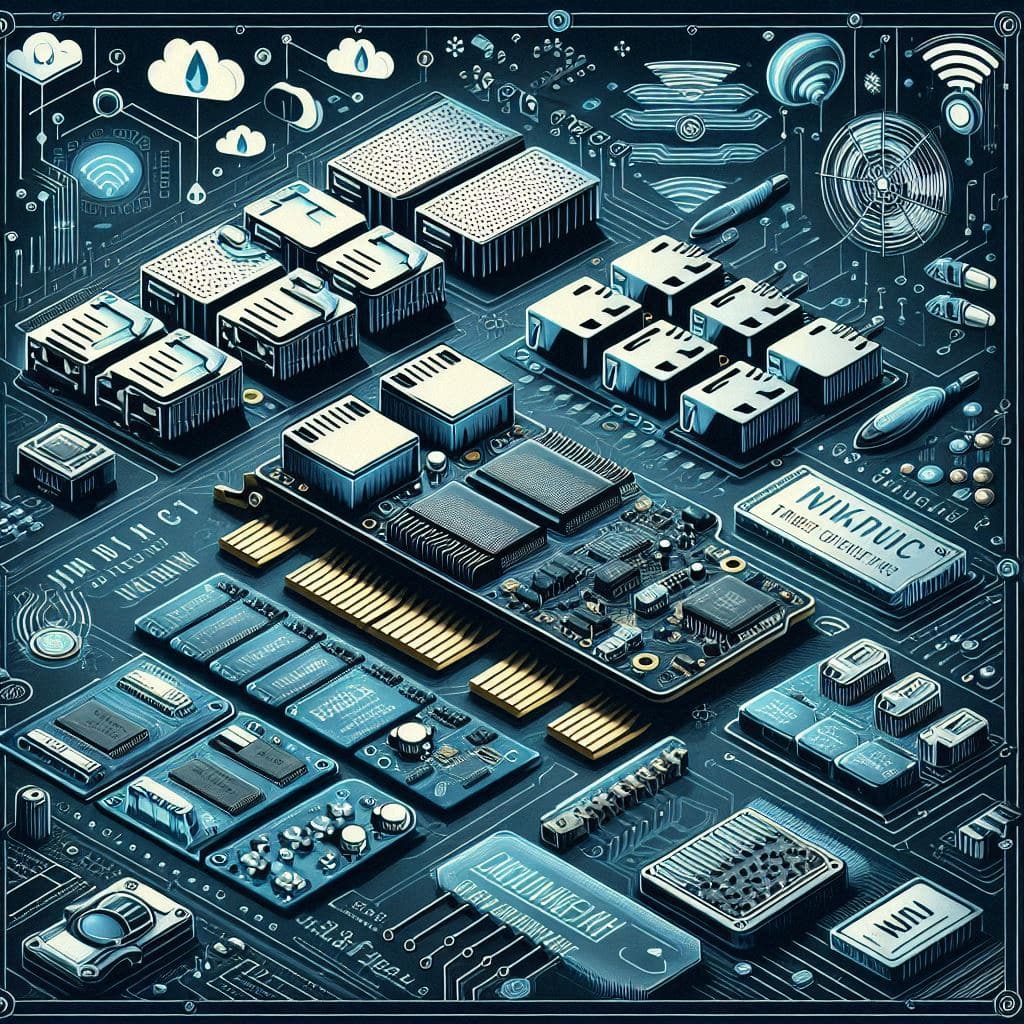
Network Cards (NICs), usually alluded to as organize cards, are basic parts in current processing gadgets. These cards act as the vital point of interaction empowering availability over both wired and remote mediums. They permit gadgets to get to neighborhood (LANs) and the web, working with proficient information move and correspondence across different applications and conditions. From individualized computing to big business level systems administration arrangements, NICs support a wide exhibit of organization conventions and assume a basic part in guaranteeing consistent combination inside computerized environments.
History and Evolution of Network Cards
Early Development and Impact
The development of organization cards follows back to the beginning of processing, where introductory endeavors were centered around associating centralized servers and terminals. The presentation of Ethernet during the 1970s by Xerox PARC denoted a critical achievement, laying the preparation for wired systems administration. The primary Ethernet NICs were created to interface gadgets over coaxial links, working at velocities of 10 Mbps. This development reformed how PCs imparted, taking into account the making of additional perplexing and productive organizations.
Advancements in Technology
As innovation progressed, so did the capacities of organization cards. The progress from coaxial links to turned pair links during the 1990s further developed network dependability and execution. The presentation of Quick Ethernet (100 Mbps) and accordingly Gigabit Ethernet (1 Gbps) NICs tended to the developing requirement for higher information move speeds. The improvement of remote systems administration advances, especially Wi-Fi, further extended the job of NICs, empowering extraordinary versatility and adaptability in network availability.
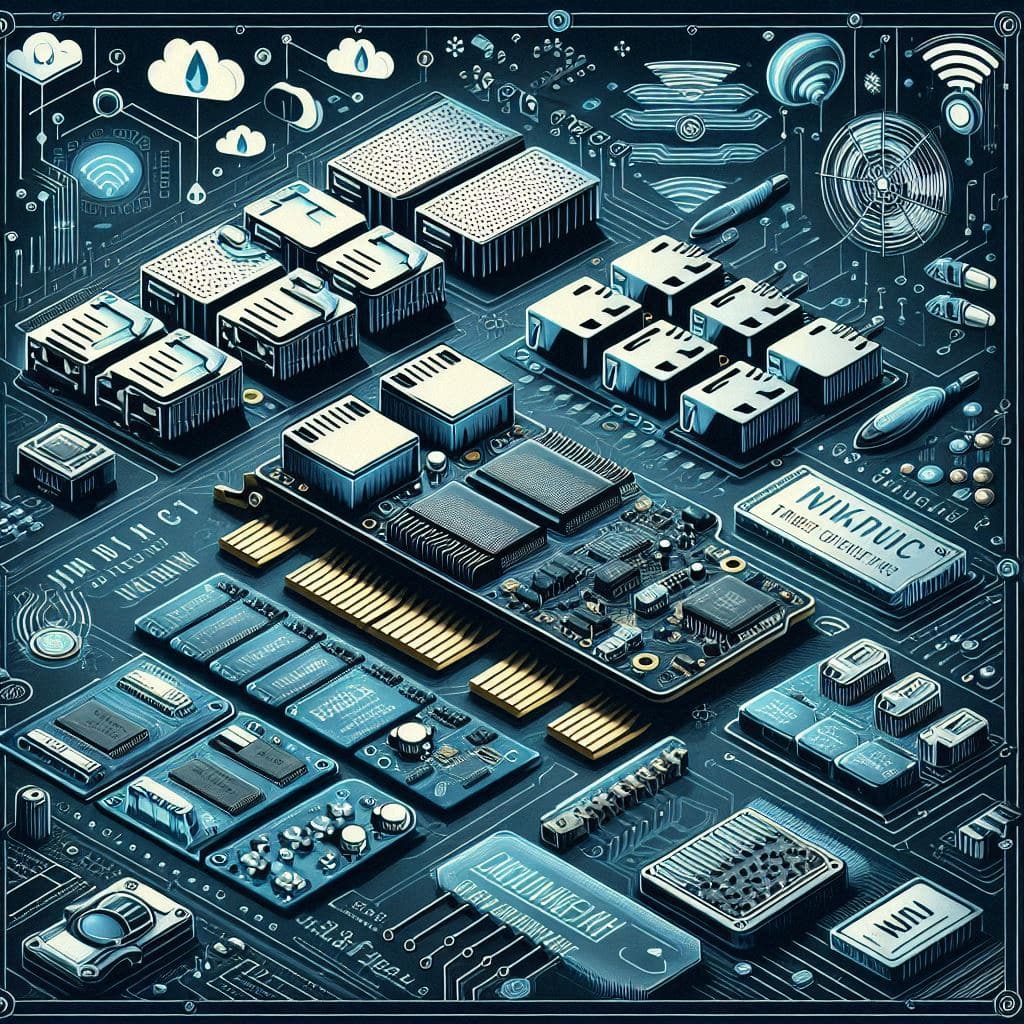
Wired Network Interface Cards (NICs)
Ethernet NICs: The Backbone of Wired Connectivity
Ethernet NICs have for some time been inseparable from dependable, rapid wired associations in processing conditions. Using Ethernet links and RJ-45 ports, these NICs guarantee soundness and hearty execution, taking special care of the rising requests for information speed and unwavering quality in current organizations.
Definition and Functionality
Ethernet NICs work with fast information moves over Ethernet links, supporting paces going from 10 Mbps to 10 Gbps relying upon network foundation and card particulars. This ability is significant for undertakings requiring steady organization execution, for example, enormous document moves, continuous video web based, and internet gaming. They support conventions like TCP/IP (Transmission Control Convention/Web Convention) and UDP (Client Datagram Convention), guaranteeing similarity across assorted network conditions.
Core Features
Present day Ethernet NICs consolidate progressed highlights, for example, auto-exchange, empowering them to consequently distinguish and design the ideal association speed and duplex mode (half or full duplex) for greatest execution. Moreover, advances like Nature of Administration (QoS) focus on basic organization traffic, guaranteeing a consistent client experience significantly under weighty organization loads or in conditions with numerous gadgets.
Limitations
In spite of their benefits in speed and dependability, Ethernet NICs are restricted by their reliance on actual associations through links. This limitation confines versatility and adaptability, especially in conditions where consistent network across various areas inside a home or office is required. Notwithstanding, innovations like Control over Ethernet (PoE) and Ethernet over Powerline (EoP) alleviate a portion of these constraints by empowering information and power transmission over existing electrical wiring, in this way upgrading organization adaptability.
Wireless Network Interface Cards (NICs)
Wi-Fi Cards: Enabling Wireless Freedom
Wi-Fi cards reform availability by empowering gadgets to interface remotely to nearby organizations and the web, using radio frequencies for information transmission. They give portability and adaptability, permitting clients to move close enough to Wi-Fi areas of interest while keeping up with consistent web access.
Introduction to Wi-Fi Cards
Wi-Fi cards support different guidelines, for example, 802.11ac (Wi-Fi 5) and the most recent Wi-Fi 6 (802.11ax), which upgrade information move speeds, increment network territory, and further develop productivity in high-thickness conditions. These principles are vital for supporting various associated gadgets all the while without compromising execution or security.
Evolution of Wi-Fi Standards
The progress from 802.11ac to Wi-Fi 6 addresses a critical headway in remote innovation, presenting highlights like MU-MIMO (Multi-Client, Different Information, Various Result) and OFDMA (Symmetrical Recurrence Division Numerous Entrance). These advancements upgrade range usage and organization proficiency, conveying quicker and more solid remote associations even in jam-packed conditions with various contending gadgets.
Technological Advancements
Present day Wi-Fi cards incorporate trend setting innovations, for example, beamforming, which upgrades signal strength and unwavering quality by centering signals towards associated gadgets. This guarantees predictable execution across shifting distances and through actual hindrances, pursuing Wi-Fi an ideal decision for private and business applications where portability and adaptability are fundamental.
Bluetooth Cards: Facilitating Short-Range Connectivity
Bluetooth cards work with short-range remote correspondence between gadgets, supporting capabilities, for example, remote sound streaming, document sharing, and network with peripherals and brilliant gadgets inside a restricted sweep.
Introduction to Bluetooth Cards
Bluetooth innovation improves gadget availability without requiring web access, making it appropriate for interfacing cell phones, PCs, and IoT gadgets like savvy speakers and wearables. Bluetooth Low Energy (BLE) variations streamline power utilization, empowering broadened procedure on battery-fueled gadgets.
Improvements in Bluetooth Technology
Ongoing cycles of Bluetooth, including Bluetooth 5.2 and then some, present upgraded highlights, for example, expanded range, higher information move rates, and further developed concurrence with other remote advances. These progressions support the dependability and adaptability of Bluetooth cards, growing their applications in shrewd homes, auto frameworks, and medical services gadgets.
Specialized Network Interface Cards
Fiber Optic NICs: Speed and Distance
Fiber optic NICs are particular organization cards intended for rapid information moves over significant distances utilizing fiber optic links. These NICs are especially common in server farms and undertaking networks where huge volumes of information should be communicated rapidly and dependably.
Definition and Functionality
Fiber optic NICs use light motions toward send information, offering essentially higher data transmission and lower dormancy contrasted with customary copper-based Ethernet NICs. They support speeds going from 1 Gbps to 100 Gbps and then some, making them ideal for elite execution organizing conditions.
Core Features
Key highlights of fiber optic NICs incorporate help for significant distance transmission without signal debasement, insusceptibility to electromagnetic obstruction, and upgraded security because of the trouble of tapping fiber optic links. These elements make fiber optic NICs appropriate for basic applications requiring fast and secure information moves.
Virtual Network Interface Cards (vNICs)
Introduction to vNICs
Virtual Organization Connection point Cards (vNICs) are programming based NICs utilized in virtualized conditions. They empower virtual machines (VMs) to interface with networks as though they had actual NICs, working with correspondence and information move inside virtualized server farms.
Functionality and Benefits
vNICs give similar functionalities as actual NICs, including support for different organization conventions and information move speeds. They offer a few advantages like adaptability in network setup, effective asset usage, and simplicity of the executives in virtualized conditions. By abstracting the organization layer, vNICs empower dynamic organization the board and versatility, essential for present day cloud and virtualized frameworks.
Technological Trends and Future Directions
Increasing Speeds and Bandwidth
The interest for higher organization speeds and more noteworthy data transfer capacity keeps on driving the improvement of cutting edge NICs. With the approach of innovations like 5G and the rising dependence on distributed computing, NICs are advancing to help quicker information moves and higher organization limits. Future NICs are supposed to offer velocities surpassing 100 Gbps, working with consistent availability for information serious applications.
Integration with IoT and Smart Devices
The multiplication of Web of Things (IoT) gadgets is impacting the plan and usefulness of NICs. Current NICs are being incorporated with IoT-explicit elements, like upgraded security conventions and energy-effective activity modes. This reconciliation guarantees that NICs can uphold the developing number of associated gadgets in brilliant homes, urban communities, and modern conditions.
Enhanced Security Features
As digital dangers become more refined, the security highlights of NICs are likewise developing. Future NICs are supposed to integrate progressed safety efforts, for example, equipment based encryption, secure boot cycles, and ongoing danger location abilities. These highlights will improve the general security of arranged gadgets, safeguarding against information breaks and digital assaults.
Application Scenarios and Use Cases
Home Networks
In home organizations, NICs assume a critical part in empowering web availability and organization correspondence between different gadgets, like PCs, cell phones, shrewd televisions, and IoT devices. Ethernet NICs give solid wired associations with fixed gadgets, while Wi-Fi and Bluetooth NICs offer remote adaptability for versatile and brilliant gadgets.
Enterprise Networks
In big business organizations, NICs are fundamental for associating servers, workstations, and systems administration hardware. Ethernet NICs are generally utilized for their speed and unwavering quality, supporting high-volume information moves and basic business applications. Fiber optic NICs are pervasive in server farms, offering rapid associations for capacity organizations and cloud administrations.
Data Centers
Server farms depend intensely on elite execution NICs to oversee immense measures of information and guarantee consistent correspondence between servers. Fiber optic NICs and high level Ethernet NICs with rates of 10 Gbps or higher are standard in server farm conditions. vNICs are additionally generally utilized in virtualized server farms, giving adaptable and versatile organization answers for virtual machines.
Industrial and Automotive Applications
In modern settings, NICs empower the availability of different machines and control frameworks, working with robotization and constant information trade. In the auto business, NICs are utilized in associated vehicles to help highlights like infotainment frameworks, route, and vehicle-to-everything (V2X) correspondence. These applications request NICs with vigorous execution and high unwavering quality.
Conclusion
Network cards, enveloping Ethernet for strong wired associations and Wi-Fi/Bluetooth for remote adaptability, structure the foundation of current availability arrangements. Their development keeps on rethinking how gadgets collaborate and convey in a carefully interconnected world. As innovation progresses, network cards will assume an undeniably essential part in upgrading efficiency, empowering consistent advanced encounters, and supporting the expansion of IoT gadgets and brilliant conditions.
All in all, the flexibility and usefulness of organization cards guarantee they stay basic parts in registering and media communications. Whether working with high velocity information moves over Ethernet or giving remote availability through Wi-Fi and Bluetooth, these cards empower people and associations to remain associated, useful, and imaginative in an always developing mechanical scene. Looking forward, progressing advancements in network card advances guarantee considerably more prominent availability, effectiveness, and dependability for clients around the world.
The proceeded with development in network cards will additionally overcome any issues among physical and virtual universes, improving our capacity to flawlessly communicate with advanced conditions. As we advance towards a more associated future, the job of organization cards will be fundamental in supporting the framework required for brilliant urban communities, independent frameworks, and then some. The excursion of NICs from their beginning to their present status highlights their significance and makes way for their vital job coming down the line for worldwide availability.
-
Blog2 years ago
Best Makeup Trends In 2024: Look Beautiful this year
-
Appliances12 months ago
Introduction to Network Cards: Evolution, Types, and Technologies
-
Blog1 year ago
Biotech Breakthroughs: Innovations in Gene Editing, CRISPR Technology, and Personalized Medicine
-
Appliances12 months ago
Intel vs. Apple Silicon: CPU Showdown for Laptops
-
Blog2 years ago
Top 5 Best Housewarming Gifts to Warm Someone’s New Home
-
Technology11 months ago
Harnessing Solar Power On-the-Go: The Rise of Foldable Solar Panel Installations
-
Technology1 year ago
The Rise of Neurotechnology: Exploring the Brain-Computer Interface
-
Appliances3 years ago
Black Friday Home Appliance Deals: Our Exclusive Sneak Peek On The Early Black Friday Appliances Deals